Contructing Explainability TRR 318
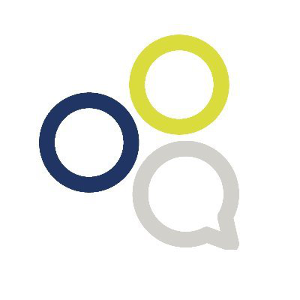
Different strategies are necessary for different situations in machine-based decision-making. The strategy to be used depends, for instance, on the amount of time or information available to make the decision. In Project C02, researchers from the fields of computer science and economics are working on a method to adapt decision-making models to different situations in which experts and users are incorporated in the process of construction. The goal is to enable decision-makers to choose the most suitable model and to be able to retroactively check the decision made.
Today, machine learning is commonly used in dynamic environments such as social networks, logistics, transportation, retail, finance, and healthcare, where new data is continuously being generated. In order to respond to possible changes in the underlying processes and to ensure that the models that have been learned continue to function reliably, they must be adapted on a continuous basis. These changes, like the model itself, should be kept transparent by providing clear explanations for users. For this, application-specific needs must be taken into account. The researchers working on Project C03 are considering how and why different types of models change from a theoretical-mathematical perspective. Their goal is to develop algorithms that efficiently and reliably detect changes in models and provide intuitive explanations to users.