Explainable Machine Learning for Interactive Episodic Updates of Models
EML4U
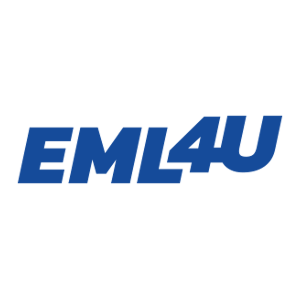
EML4U
In this BMBF project, methods in the area of explainability and transparency of machine learning are further developed. These should make it possible to answer a new and central question for episodic interactive learning: Given new data, is an update of an ML model necessary, and if so, how can the likely effects of post-training be explained?
Machine Learning (ML) allows complex relationships to be modeled using data. Thus, complex and often grossly simplified mathematical modelling of certain conditions can be avoided. In addition, a new type of functionality opens up: ML models can be adapted to changing requirements and conditions in a data-driven manner. In order to achieve a regular adaptation, data is collected during the use of a model and the model is re-trained taking this information into account. Thus, an episodic update of the ML model takes place.